9 Constraints Visual Object Recognition

9 Constraints Visual Object Recognition Youtube Mit 6.034 artificial intelligence, fall 2010view the complete course: ocw.mit.edu 6 034f10instructor: patrick winstonwe consider how object recognitio. Lecture 9: constraints: visual object recognition description: we consider how object recognition has evolved over the past 30 years. in alignment theory, 2 d projections are used to determine whether an additional picture is of the same object.
Constraints Visual Object Recognition Coggle Diagram Together, these findings illustrate how the human visual system is not a ‘blank slate’ at birth, but is already equipped with many biases and constraints that support object recognition. Figure 1. core object recognition. core object recognition is the ability to rapidly (<200 ms viewing duration) discriminate a given visual object (e.g., a car, top row) from all other possible visual objects (e.g., bottom row) without any object specific or location specific pre cuing (e.g., dicarlo and cox, 2007). Visual object recognition has been one of the main subfields of cognitive neuroscience to first adapt these methods 53. object recognition is fast 5; we can recognize an object in tens of milliseconds. this is much faster than the typical resolution of bold fmri (e.g. 2 seconds); thus, unpacking the temporal evolution of object representations. (a) the response pattern of a population of visual neurons (e.g., retinal ganglion cells) to each image (three images shown) is a point in a very high dimensional space where each axis is the response level of each neuron. (b) all possible identity preserving transformations of an object will form a low dimensional manifold of points in the population vector space, i.e., a continuous surface.
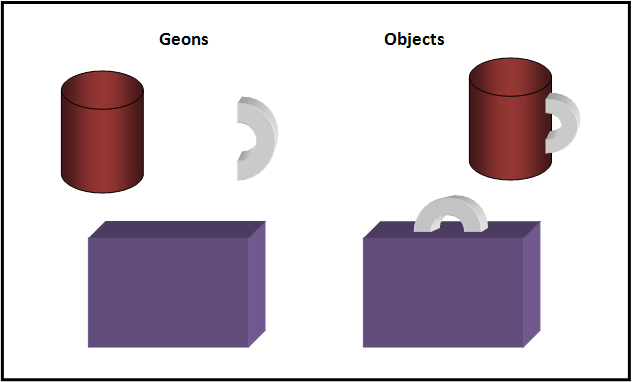
Cognitive Neuroscience Of Visual Object Recognition Psynso Visual object recognition has been one of the main subfields of cognitive neuroscience to first adapt these methods 53. object recognition is fast 5; we can recognize an object in tens of milliseconds. this is much faster than the typical resolution of bold fmri (e.g. 2 seconds); thus, unpacking the temporal evolution of object representations. (a) the response pattern of a population of visual neurons (e.g., retinal ganglion cells) to each image (three images shown) is a point in a very high dimensional space where each axis is the response level of each neuron. (b) all possible identity preserving transformations of an object will form a low dimensional manifold of points in the population vector space, i.e., a continuous surface. Borders and faces orientation discerning borders of objects and face orientation with vectors, an initial computer vision theory seemed plausible but too difficult to implement. orthographic projection in orthographic projection, the correspondence of a system of points of three known objects and one unknown object creates a system of equations with a unique solution of … continue reading "9. Course in various techniques and theories within object recognition, detection, tracking, segmentation etc. including: invariant local features and feature extraction in digital images, bag of features framework, principles of object recognition and detection, local spatial constraints, introduction to convolutional neural networks, support vector machines learning, shape descriptors and.
Github Viktorgus Visual Object Recognition And Detection Tsbb17 Borders and faces orientation discerning borders of objects and face orientation with vectors, an initial computer vision theory seemed plausible but too difficult to implement. orthographic projection in orthographic projection, the correspondence of a system of points of three known objects and one unknown object creates a system of equations with a unique solution of … continue reading "9. Course in various techniques and theories within object recognition, detection, tracking, segmentation etc. including: invariant local features and feature extraction in digital images, bag of features framework, principles of object recognition and detection, local spatial constraints, introduction to convolutional neural networks, support vector machines learning, shape descriptors and.
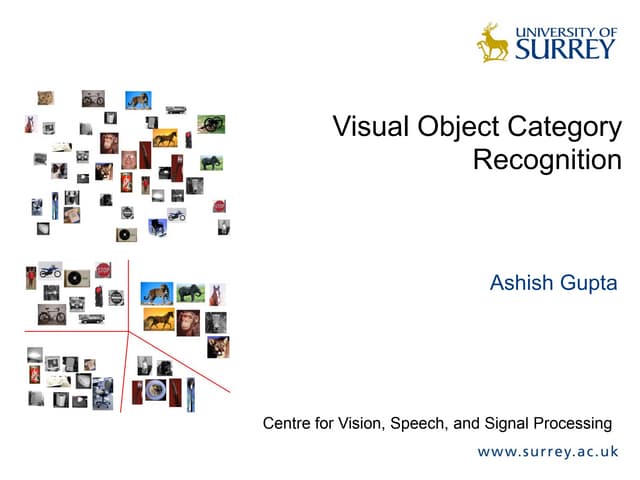
Visual Object Category Recognition Ppt

What Is Visual Object Recognition Josephine Wilson S Sight Words
Comments are closed.