Sensor Fusion For Autonomous Vehicles Strategies Methods And Tradeoffs Synopsys
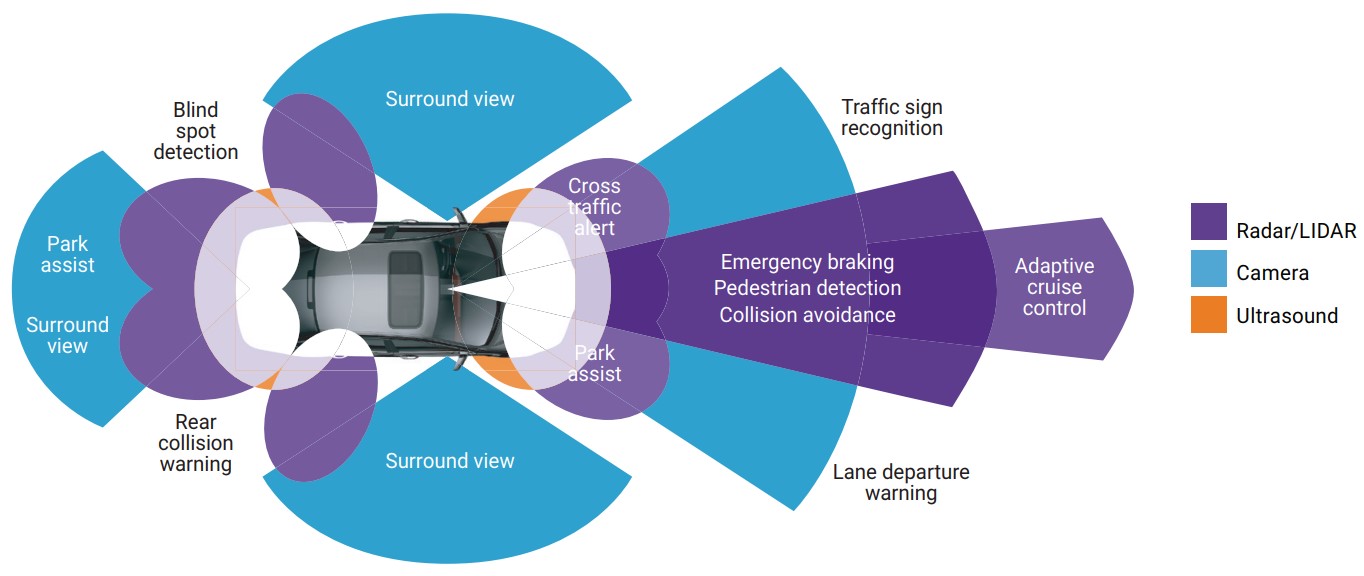
Multi Sensor Fusion For Robust Device Autonomy This video presents key sensor fusion strategies for combining heterogeneous sensor data in automotive socs. it discusses the three main fusion methods that. Sensor fusion vs. edge computing. sensor fusion is the process of combining data from multiple different sensors to generate a more accurate “truth” and help the computer make safe decisions even though each individual sensor might be unreliable on its own. in the case of autonomous vehicles, signals from a wide variety of sensors are.

Process In Sensor Fusion Mechanism In Autonomous Vehicle Download Sensor fusion technology is a critical component of autonomous vehicles, enabling them to perceive and respond to their environment with greater accuracy and speed. this technology integrates data from multiple sensors, such as lidar, radar, cameras, and gps, to create a comprehensive understanding of the vehicle’s surroundings. by combining and analyzing this data, sensor fusion technology. The current paper, therefore, provides an end to end review of the hardware and software methods required for sensor fusion object detection. we conclude by highlighting some of the challenges in the sensor fusion field and propose possible future research directions for automated driving systems. 1. Although autonomous vehicles (avs) are expected to revolutionize transportation, robust perception across a wide range of driving contexts remains a significant challenge. techniques to fuse sensor data from camera, radar, and lidar sensors have been proposed to improve av perception. however, existing methods are insufficiently robust in difficult driving contexts (e.g., bad weather, low. We also summarize the three main approaches to sensor fusion and review current state of the art multi sensor fusion techniques and algorithms for object detection in autonomous driving.
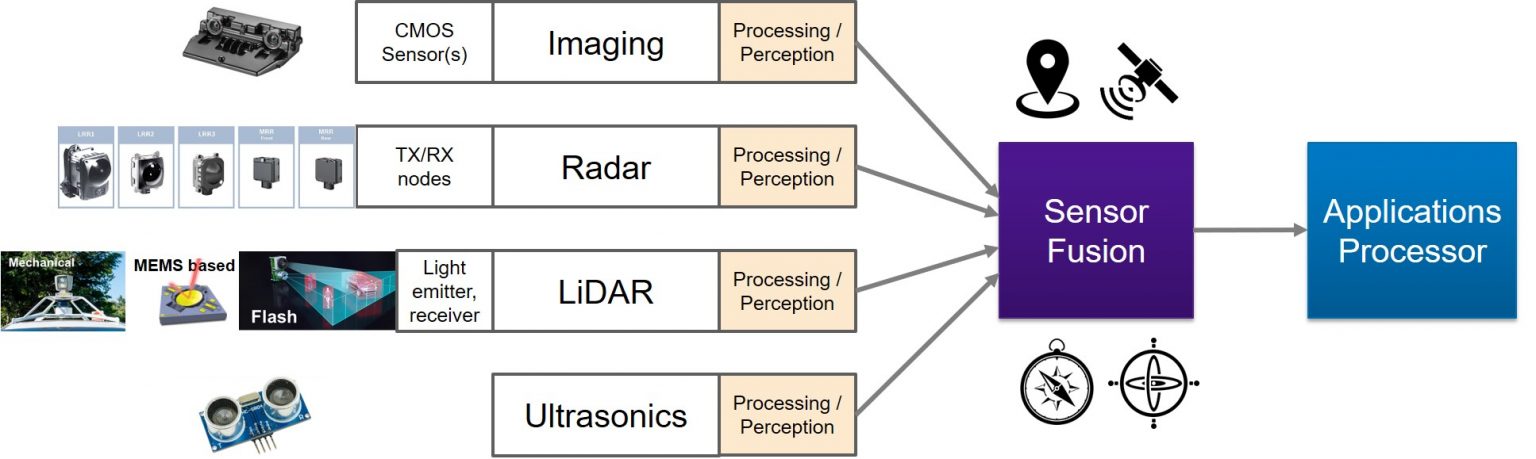
Multi Sensor Fusion For Robust Device Autonomy Edge Ai And Vision Although autonomous vehicles (avs) are expected to revolutionize transportation, robust perception across a wide range of driving contexts remains a significant challenge. techniques to fuse sensor data from camera, radar, and lidar sensors have been proposed to improve av perception. however, existing methods are insufficiently robust in difficult driving contexts (e.g., bad weather, low. We also summarize the three main approaches to sensor fusion and review current state of the art multi sensor fusion techniques and algorithms for object detection in autonomous driving. For ad applications, lidar sensors with 64‐ or 128‐ channels are commonly em‐ployed to generate laser images (or point cloud data) in high resolution [61,62]. 1d or one‐dimensional sensors. The tracking accuracy of nearby vehicles determines the safety and feasibility of driver assistance systems or autonomous vehicles. recent research has been active to employ additional sensors or to combine heterogeneous sensors for more accurate tracking performance. especially, autonomous driving technologies require a sensor fusion technique that considers various driving environments. in.

Sensor Fusion Challenges In Cars For ad applications, lidar sensors with 64‐ or 128‐ channels are commonly em‐ployed to generate laser images (or point cloud data) in high resolution [61,62]. 1d or one‐dimensional sensors. The tracking accuracy of nearby vehicles determines the safety and feasibility of driver assistance systems or autonomous vehicles. recent research has been active to employ additional sensors or to combine heterogeneous sensors for more accurate tracking performance. especially, autonomous driving technologies require a sensor fusion technique that considers various driving environments. in.
Comments are closed.